Multi System operator
Churn Prediction
Problem
The client faced mounting pressure to retain customers and needed a solution to identify early signs of customer attrition and respond proactively.
Solution
An AI-driven Logistic Regression model was developed to estimate the probability of customer attrition, utilizing the attrition flag and other key factors as independent variables.
Outcome
The model accurately identified a significant portion of customers at risk of attrition, empowering the team to create and execute targeted retention campaigns for this high-priority group.
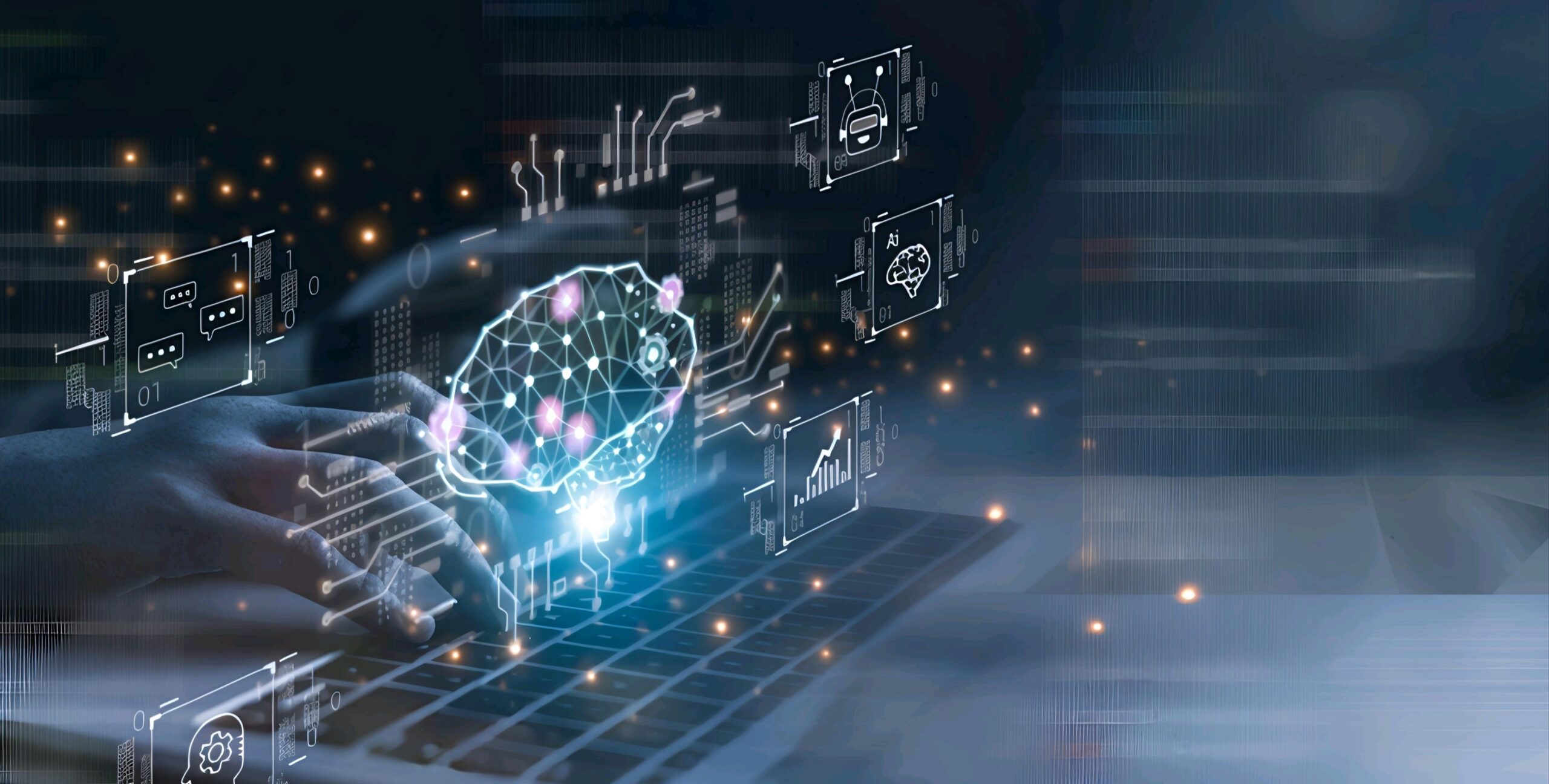
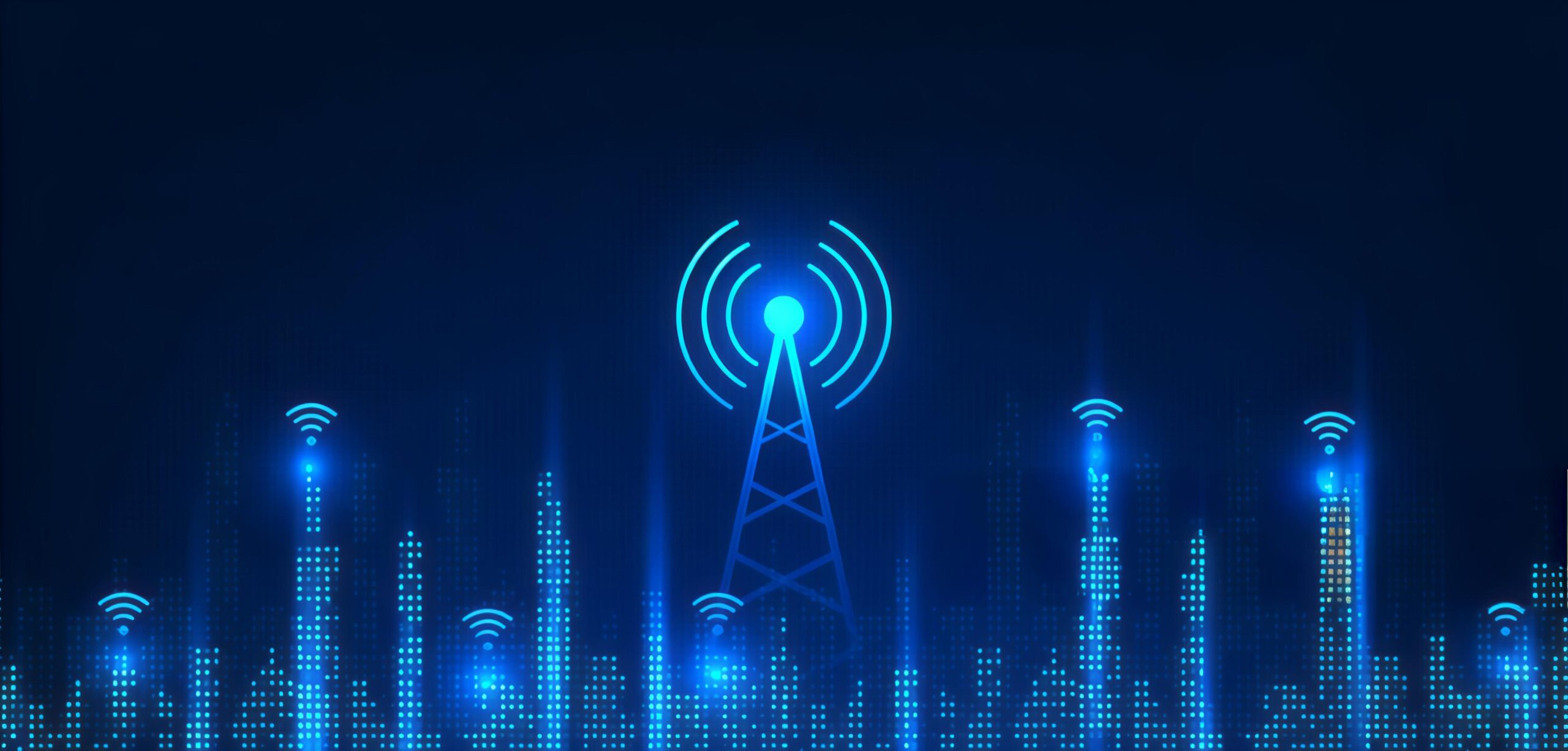
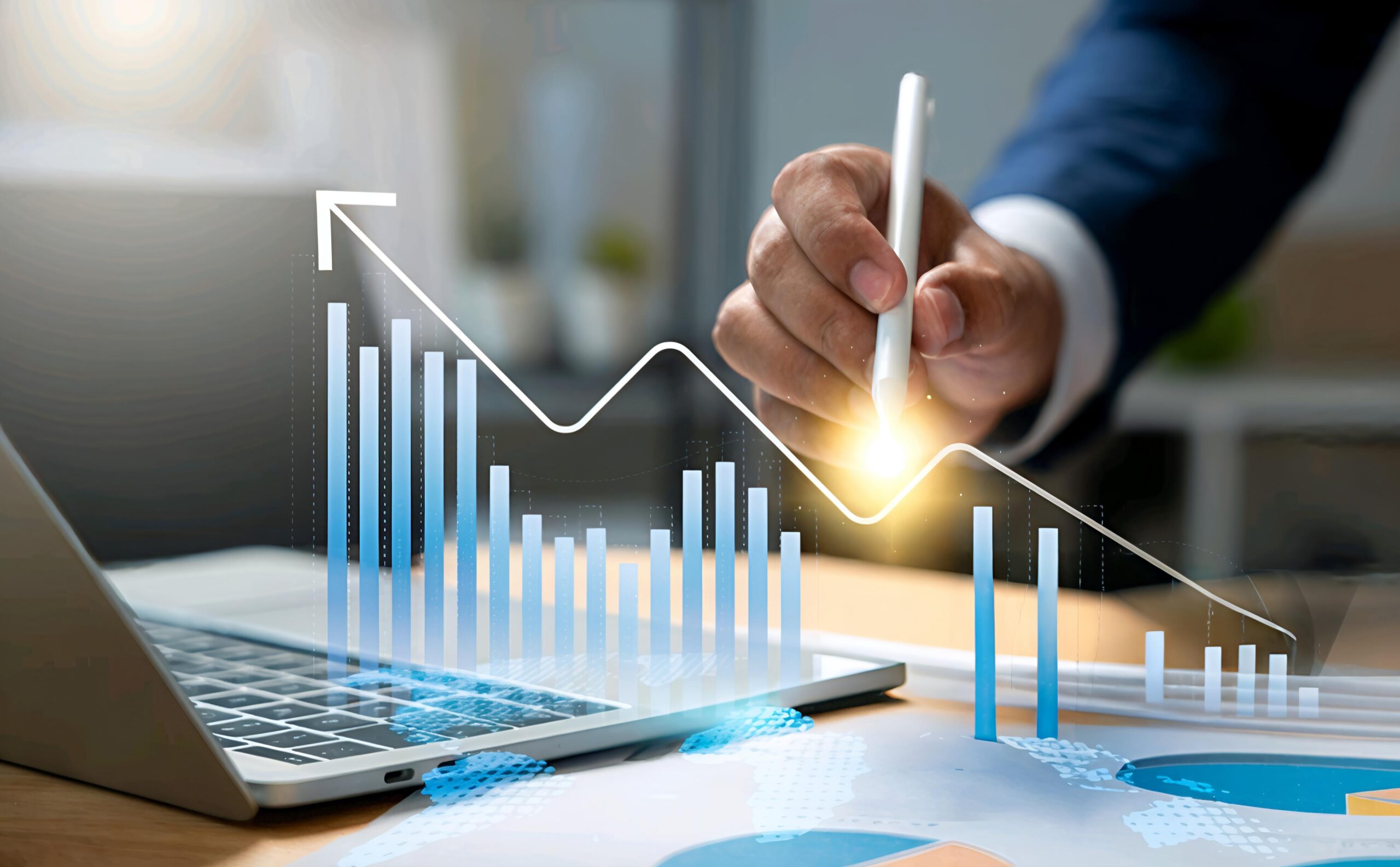
Predictive AI to uncover early signs of customer attrition
The client faced increasing challenges in retaining customers. To address this, they sought a solution capable of identifying early signs of customer attrition, enabling the creation of more targeted retention strategies. Viewership behavior, subscription patterns, customer interactions, and payment histories were identified as critical factors influencing retention. A Logistic Regression model was developed using two-year-old customer data, with the attrition flag as the dependent variable and other attributes as predictors.
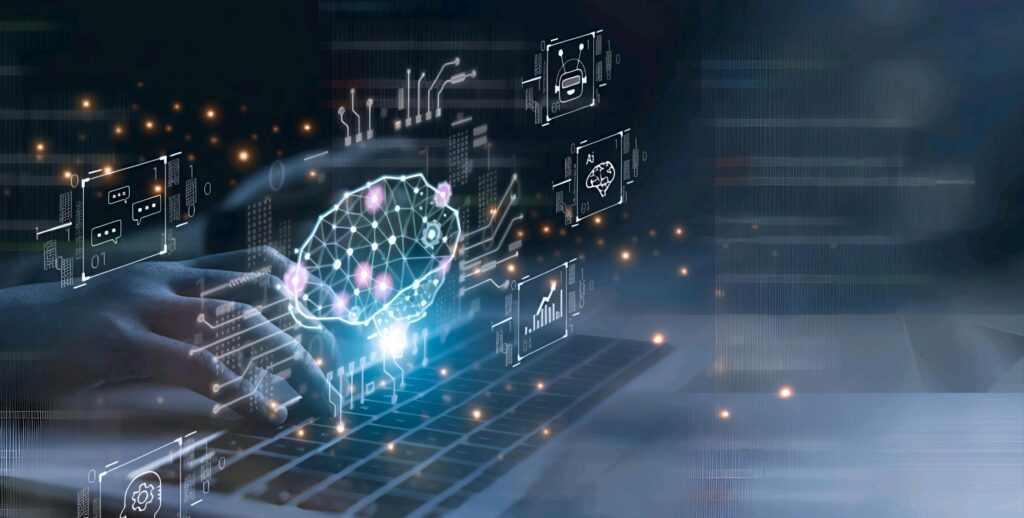
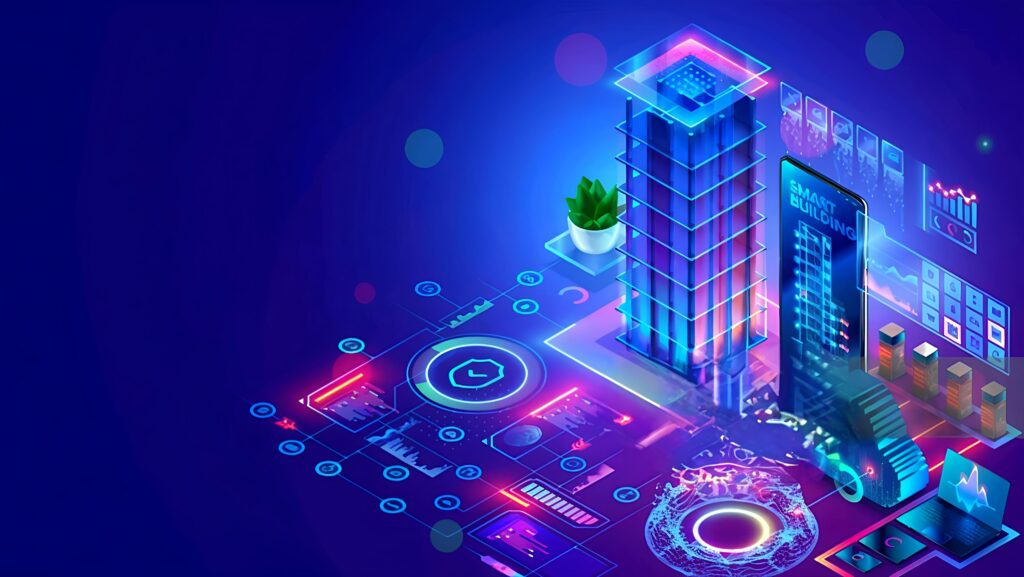
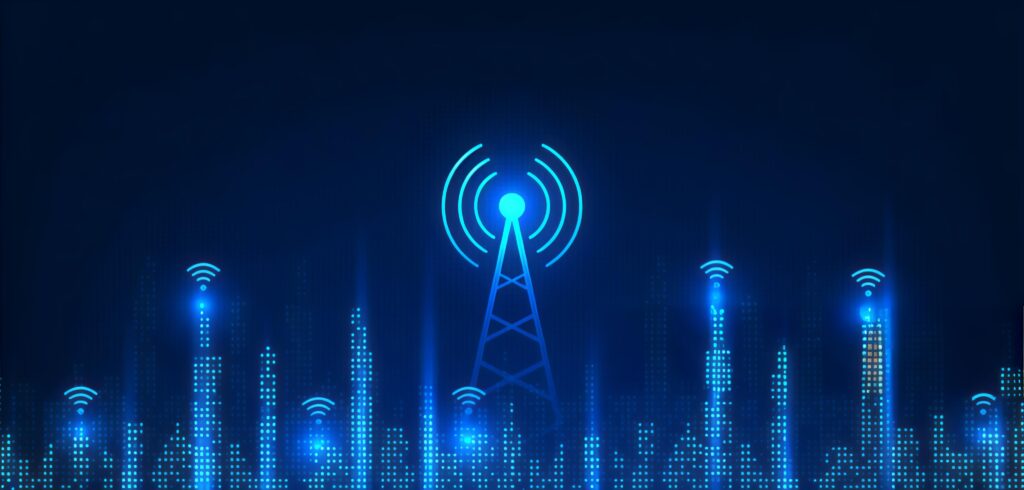
The model achieved a prediction accuracy of 97% and identified approximately 13%, or 1.5 million, of the customer base as potential attrition candidates. Further analysis revealed higher attrition risks in urbanized states like New York and California. Leveraging these insights, the client’s marketing team designed targeted campaigns that effectively addressed the retention challenges.
Saving Subscribers with AI
Explore All
Discover our complete range of high-quality products designed to meet your needs. Explore now and find the perfect fit for your requirements.